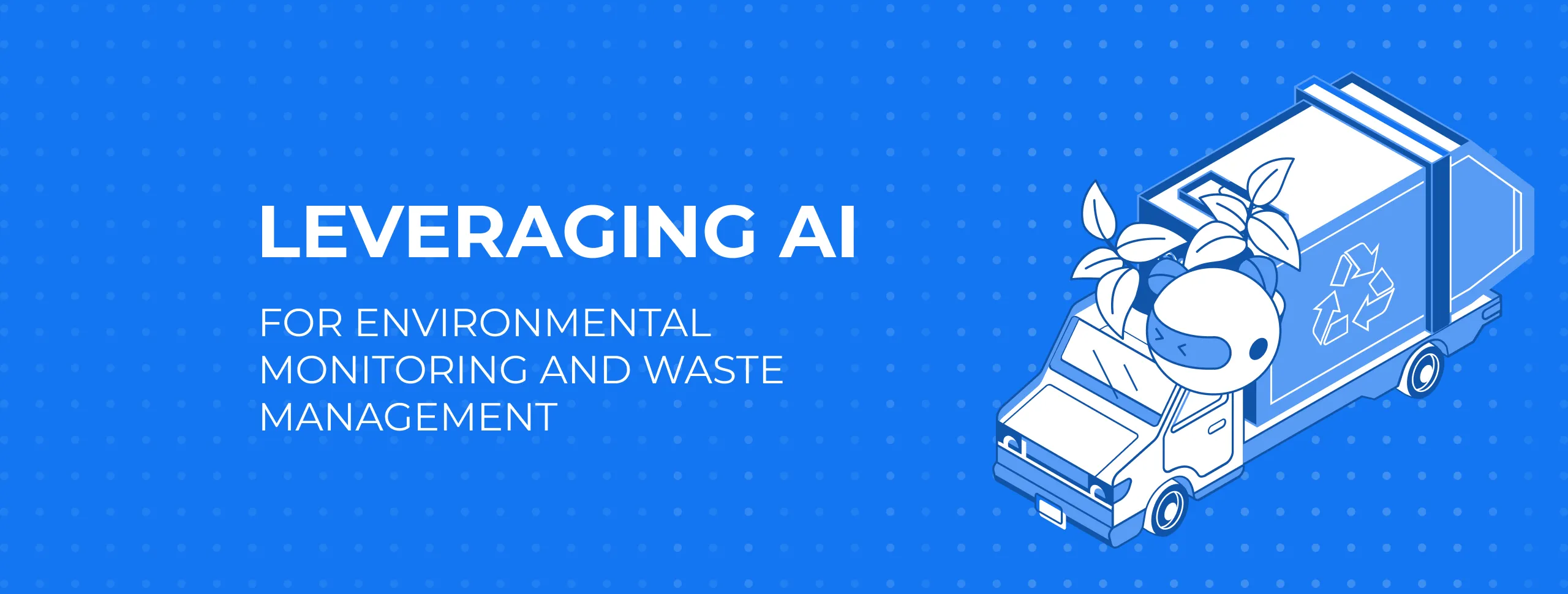
3 Ways AI is Shaping the Future of Sustainability
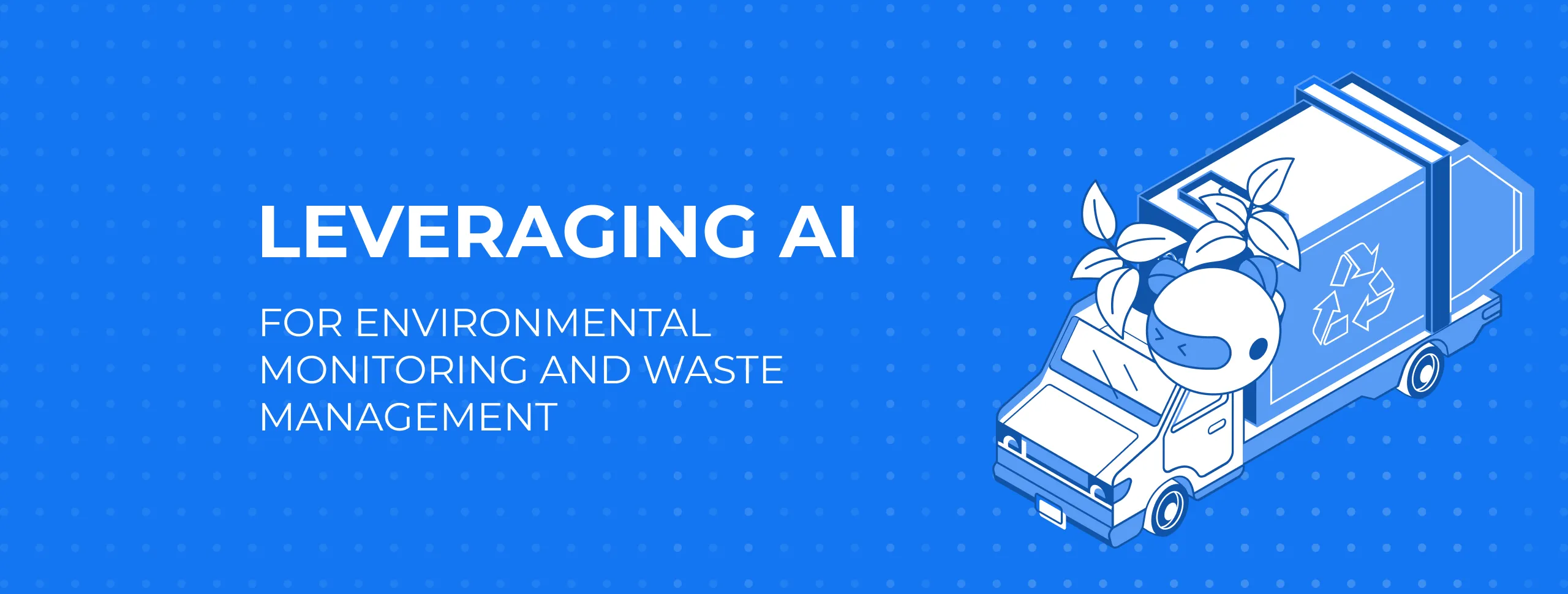
Table of contents
- The Importance of Environmental Monitoring in Urban Areas
- AI in Sustainability: Air Quality Monitoring
- AI in Sustainability: Water Quality Monitoring
- AI in Sustainability: Waste Management
- Benefits and Challenges of Implementing AI in Cities
- AI in Sustainability: Final Thoughts
With the rapid boom of Artificial Intelligence (AI), it’s now evident that this new technology will dramatically impact every aspect of life. And while a lot has been said about the ways it could influence sectors like healthcare, education, real estate and hospitality, there’s less discussion around the intersection of AI and sustainability.
Will AI play an important role in the fight against climate change? That’s quite likely, according to a recent study by PwC UK. According to their research, AI applications in sectors such as agriculture, water, energy, and transport could add up to $5.2 trillion USD to the global economy by 2030, a 4.4% increase compared to current projections.
The study also found that AI could help cut global greenhouse gas emissions by 4%, or 2.4 Gt CO2e. To put that in context, this is the equivalent to the combined annual 2030 emissions of Australia, Canada, and Japan.
The Importance of Environmental Monitoring in Urban Areas
One key aspect where AI can significantly contribute to sustainability is environmental monitoring. Environmental monitoring uses sensors, wireless connectivity, edge computing, and visualization tools to track environmental health and detect potential issues.
A broad term encompassing areas such as air and water quality monitoring, and waste management, the effectiveness of environmental monitoring greatly impacts urban regions. Here’s why robust environmental monitoring is crucial:
Public Health
Environmental monitoring, and air quality monitoring in particular, is crucial for public health. According to the World Health Organization (WHO), nearly 7 million premature deaths happen every year due to air pollution.
Climate Change Mitigation
Urban areas are responsible for more than 70% of global greenhouse gas emissions. That’s why, in order to fight climate change—which is one of today’s biggest threats—environmental monitoring is a must.
Urban Heat Islands
Perhaps you’ve heard of the term Urban Heat Islands (UHI). It means that the temperature is often higher in the cities than in rural areas. That’s because human-made structures like buildings and roads absorb and reemit heat more than natural landscapes. The UHI effect is bad for both public health and the environment – it is linked to heat-associated health risks and higher energy consumption.
Disaster Preparedness
Environmental monitoring tracks natural hazards like floods, landslides, and extreme weather events. Thanks to the data it produces, people living in cities can be better prepared to address such events.
Biodiversity Protection
Monitoring urban biodiversity helps in maintaining and restoring natural habitats within cities. This is important because it supports the conservation of local species and ensures ecological balance.
Let’s take a closer look at how AI supports sustainability in the different areas of environmental monitoring.
Created with Dall-E.
1. AI in Sustainability: Air Quality Monitoring
Air quality monitoring refers to the measurement of different air pollutants, also known as the “criteria level pollutants” (particulate matter (PM10 and PM2.5), ozone (O3), nitrogen dioxide (NO2), sulfur dioxide (SO2), carbon monoxide (CO), and lead (Pb)).
There are three key technologies used for air quality monitoring, and they all take significant advantage of AI: air quality sensors, satellite data and remote sensing, and predictive analytics.
Air Quality Sensors
Air quality sensors use optical or electrochemical sensors to detect pollutants.
Optical sensors emit light into the air and measure the scattering or absorption caused by particles. Electrochemical sensors, on the other hand, detect gases based on chemical reactions.
How does AI help?
AI helps to assess and manage air quality in real time. In this way, governments and scientists can continuously monitor pollutants with unprecedented accuracy.
Real-life example:
Thanks to AI, scientists now accurately measure PM2.5 levels in New York City. Previously, the researchers had to rely on complex models that needed extensive data inputs, which was making them hard to use.
Engineers at Cornell University came up with a simplified model that is easier to use. They leverage AI to gain a detailed view of street air pollution, and PM2.5 levels in particular. Coming from traffic exhaust, this type of pollution is detrimental to human health. By combining traffic data, topology and meteorology, the AI algorithm simulates and predicts traffic-related air pollution concentrations.
Satellite Data and Remote Sensing
Satellite-based remote sensing uses complex tools like spectrometers and radiometers to measure the atmospheric composition from space. These instruments capture data across various wavelengths of light, which allows them to detect and quantify pollutants, aerosols, and greenhouse gases.
How does AI help?
AI algorithms process the massive volumes of spectral data that satellites collect. They also analyze the data to identify patterns, trends, and correlations in air quality metrics much more efficiently than traditional methods. In this way, scientists can rapidly assess and manage air quality on a global scale.
Created with Dall-E.
Real-life example:
The European Space Agency's (ESA) Copernicus project launched a new satellite called "Sentinel-5P," which can measure various pollutants and share the data publicly.
A scientific paper explores a method to combine satellite data with pollution measurements, showing that simpler models can work just as well as advanced neural networks. A new dataset was created with pollution measurements from stations across Europe, including factors like altitude, population density, environmental classification, and satellite data from the ESA project.
This dataset trains the Air Quality Network (AQNet) model, which uses these different data sources to predict pollutants.The machine learning model successfully predicts three pollutants: NO2, O3, and PM10. These predictions are combined into an "air quality index" to compare air quality across regions.
AQNet performs better than a model using only satellite images. According to the paper, testing the AI model with data from the UK and Ireland gave satisfactory results, though pollution levels were generally overestimated by about 20%.
Using Historical Data for Predictive Analytics
AI algorithms can use extensive historical datasets, including air pollutant levels, meteorological conditions, traffic patterns, and industrial activities. By analyzing this data, AI can recognize patterns and correlations and in this way predict air quality variations over time. For example, AI models can predict seasonal spikes in particulate matter (PM2.5) levels based on historical trends and weather conditions.
Real-Life Example:
UNEP's World Environment Situation Room (WESR), which was established in 2022, is a digital platform that uses AI's capabilities to analyze complex datasets. Using earth observation and sensor data, WESR supports near real-time analysis and predictions on a number of factors, including the concentration of carbon dioxide in the atmosphere.
2. AI in Sustainability: Water Quality Monitoring
Water quality monitoring involves regularly testing water sources, such as rivers and lakes, to assess their condition and identify any contaminants.
This process is crucial because clean water is essential for human health, agriculture, industry, and the environment. By monitoring water quality, cities can detect pollutants, track changes over time, and identify potential hazards before they become serious problems. This information helps in making informed decisions about water management, protecting ecosystems, ensuring safe drinking water, and preventing waterborne diseases.
How does AI help?
AI enhances water quality monitoring by analyzing large datasets from various sources such as sensors, satellites, and historical data. AI algorithms can detect changes in water quality parameters (e.g. pH levels) and the presence of contaminants such as heavy metals and harmful bacteria. With this real-time analysis, scientists can detect pollution events early and take proactive measures to protect water resources.
Real-life examples:
In practice, AI has been successfully implemented in various case studies:
- AI-powered Autonomous Underwater Vehicles (AUVs): These vehicles are equipped with sensors and AI algorithms to monitor water quality parameters in lakes, rivers, and oceans. A team of U.S. National Science Foundation-funded researchers at the Minnesota Interactive Robotics and Vision Laboratory is developing advanced AUVs to collect data, provide insights into species distribution, and create habitat maps. These innovations, tested on the MeCO platform with multiple sensors, aim to identify and track invasive species in Minnesota lakes. This project aims to make underwater robots more effective for conservation, enhancing the protection of underwater environments.
- Aquabyte's AI-powered Fish Health Monitoring: Startup Aquabyte uses AI and computer vision to monitor fish health in aquaculture farms. By analyzing images of fish, AI can detect diseases early, optimize feeding practices, and reduce environmental impacts associated with fish farming.
3. AI in Sustainability: Waste Management
Waste management refers to the collection, transportation, disposal, and recycling of waste materials generated by humans. Effective waste management in urban areas is crucial for public health, environmental sustainability, and the efficient use of resources.
Traditional waste management systems often face challenges such as inefficient collection routes, overfilled landfills, and inadequate recycling rates. These systems may struggle with optimizing resource allocation, managing diverse types of waste, and minimizing environmental impacts like air and water pollution.
Created with Dall-E.
How Does AI Help?
Cities and companies across the world leverage AI to optimize waste collection and improve recycling practices. This brings improved operational efficiency and reduced costs while enhancing environmental sustainability.
Let’s take a look at two examples:
Real-World Applications of AI in Waste Management:
- Waste Collection Optimization in the City of Barcelona, Spain:
Traditional waste collection systems usually follow a fixed schedule, which results in overflowing bins and inefficient collection routes. AI can improve the process of waste collection by using sensors to monitor bin fill levels, and then enabling automatic scheduling of pickups.
In Barcelona, Spain, AI-driven waste management systems have decreased waste collection costs by 40% and boosted recycling rates by 6%.
- Better Recycling with Waste Robotics: Waste Robotics, a company based in Canada, uses AI and robotics to automate the sorting of recyclable materials in recycling facilities. Their technology improves sorting accuracy and efficiency, leading to higher recycling rates and cleaner recycling streams.
Benefits and Challenges of Implementing AI in Cities
From the examples above, it’s evident that AI can greatly enhance environmental monitoring. Here are the key benefits of AI in sustainability:
Benefits of AI in Sustainability:
- Improved Efficiency: AI algorithms improve the processes of air quality and water quality monitoring and waste collection, which leads to much better efficiency;
- Cost Savings: By optimizing operations and reducing resource waste, AI can lower operational costs. This is great for the bottom lines of environmental monitoring companies and also improves the allocation of public resources;
- Climate Change Mitigation: AI helps in making data-driven decisions that promote sustainability, such as reducing carbon emissions through optimized transportation routes and improving recycling rates. This is key in the fight against climate change.
- Enhanced Quality of Life: Cleaner environments and efficient waste management contribute to better living conditions and improved public health.
And while AI brings a lot of benefits in urban sustainability, it does not come without challenges:
Challenges of Implementing AI in Cities:
- Data Privacy Concerns: Handling large volumes of data raises concerns about privacy and data security for residents and businesses.
- High Initial Costs: Setting up AI infrastructure, including sensors, data analytics platforms, and machine learning models, usually comes with a significant upfront investment.
- Lack of Technical Expertise: Given that AI is a nascent technology, there is still insufficient technical expertise in the area.
Strategies to Overcome These Challenges:
To address these challenges, cities can consider the following strategies:
- Data Governance and Transparency: Urban areas should implement clear policies and frameworks for data governance to ensure transparency and protect privacy.
- Public-Private Partnerships: In order to make AI technology more affordable, cities can collaborate with private sector partners to share costs, expertise, and infrastructure for AI implementation.
- Capacity Building: It’s key to invest in training programs and initiatives in order to build local technical expertise in AI and data science.
AI in Sustainability: Final Thoughts
It’s clear that AI is already starting to make a substantial impact in the field of sustainability. It has great potential to boost the efficiency of air and water quality monitoring and optimize waste collection and recycling processes.
For companies in the field of environmental monitoring, now is the time to implement AI in order to cut costs, improve efficiency and gain a competitive advantage.
Created with Lexica.art.
Our experts will support you in building AI models tailored to your specific needs. We specialize in developing customized AI solutions and ensure that every aspect of the model is aligned with your operational goals. We will also guide you through the product discovery phase to make sure your project is on the right track from the very beginning. In addition, Magora offers seamless integration of ChatGPT or similar LLM models into existing software products, enhancing their capabilities with advanced AI features.
With Magora, you can also connect the AI models with different devices and sensors, enabling real-time data processing and analysis. This integration ensures that your products are smarter, more efficient, and capable of delivering superior performance and insights. Contact us to learn more.