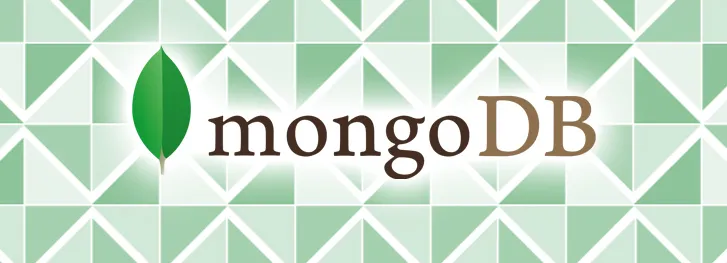
MongoDB: a Fast and Easy Way to Calculate Aggregated Values without Map-Reduce
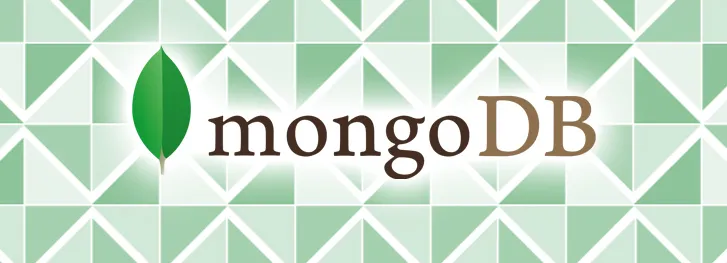
A MongoDB aggregation framework allows you to calculate aggregated values without having to use map-reduce. While map-reduce is a powerful tool, it often proves to be slow when processing big volumes of data. In this article, I would like to compare map-reduce with MongoDB and show the significant benefits of using the latter.
MongoDB vs Map-Reduce
The main differences of Aggregation Framework from Map-Reduce are:
-
declarative syntax, no need to write code in JavaScript;
-
describing chains of operations to apply;
-
expressions evaluation;
-
higher performance because aggregation framework is implemented in C++ instead of JavaScript;
-
projections of returned data so a user can add computed fields, sub-objects, etc.
Framework concepts
Aggregation Framework provides the similar logic as the “GROUP BY” SQL operator. There are 2 main concepts in aggregation framework: pipelines and expressions. Pipelines are operators that can process a stream of the documents. Expressions return the output documents after the calculations on input documents. Some pipelines:
-
$match – uses query predicate like collection.find({});
-
$project – allows to change the shape of the result, include computed values, sub-objects, etc.;
-
$unwind – separates elements of an array and add it into an output document;
-
$sort – sorts documents;
-
$limit – specifies maximum number of documents to be returned;
-
$skip – skips a specified number of documents.
Using MongoDB in Node.JS: our hands-on experience
MongoDB has drivers for many programming languages and platforms, including Node.JS. You can install Node.JS driver by typing npm install mongodb.
All MongoDB features are available in the driver. There was a task to aggregate huge data collection by three fields to build some statistical report. The collection contained about 500k records with web pages views statistics. Each document had the following format:
It was necessary to group data by time, IP address and URL. The first version of this logic was implemented using map-reduce:
The processing of 500k records took about 1 minute. It was an annoying issue and we decided to switch to the MongoDB 2.1. aggregation framework. The new version of aggregation logic is presented below:
In this code, we use 2 pipelines: $match and $group. The $match filter required records, and the $group aggregates records by three fields: time, URL and IP. These fields are used as a key because we explicitly specified ‘_id’ field and expression $sum calculates the number of records with the same key. The output data has the following view:
Result
The use of an aggregation framework significantly improved the performance of the processing. Now 500k of records are processed within 3-4 seconds. The MongoDB aggregation framework is a powerful, simple and lightweight tool that really allows you to improve the performance of aggregated values calculations without using map-reduce.